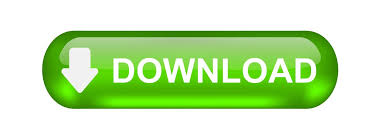
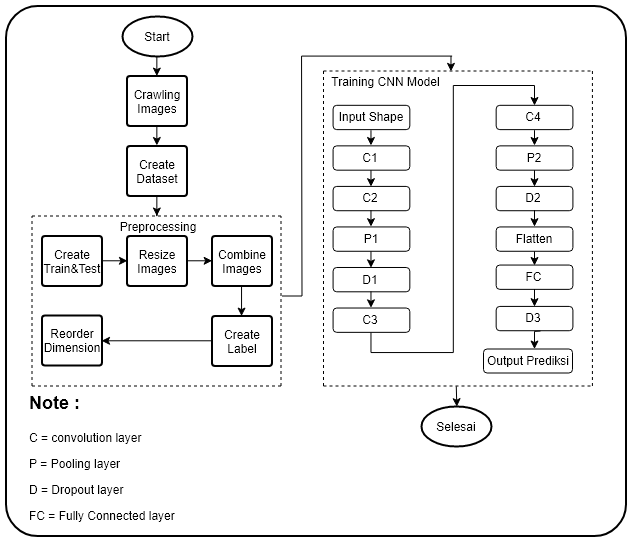
Besides, the multi-layer perceptron (MLP) is constituted by perceptron, which is a fundamental structure for the feedforward neural network (NN), in VLSI (very-large-scale integration) implementations incorporating various learning algorithms, thus making MLP a common choice as it has been continuously researched for many years. Many digital- or analog-implemented circuits for perceptron have been proposed in the literature, showing good results in the simulation phase, however the drawback of these works is the lack of silicon measurement results, which are of a great importance for the investigation of the fundamental characteristics of a perceptron circuit. ANN-based machine learning fits the transfer function of the system by a training process where input-output pairs are iteratively presented, while the variable parameters/weights are adjusted with the process. It is commonly represented by a mathematical model as follows: Each input is multiplied by a weight and all weighted inputs are summed at the output, the resulting sum is then passed through an activation function. Perceptron is an essential element of ANN, which has multiple inputs and a single output. The structure of an artificial neuron mimics the function of dendrites, soma, and axon. ANN has a huge set of neurons that are highly interconnected and arranged in layers. ANN is inspired by the brain of living creatures, which contains components such as neurons, connections, weights, and a propagation function. Finally, to demonstrate the feasibility and effectiveness of our analog perceptron for configuring a MLP, seven more analog-based MLPs designed with the same approach are used to analyze the simulation results with respect to various specifications, in which two cases are used to compare to their digital counterparts with the same structures.Īrtificial neural network (ANN)-based machine learning, known as a promising technology, has been researched widely to enable electronic devices more intelligent and efficient. Its experimental case shows that the simulated performance achieves a power dissipation of 200 mW, a range of working frequency from 0 to 1 MHz, and an error ratio within 12.7%. The example given is to design a 2-3-4 MLP circuit with rectified linear unit (ReLU) activation, which consists of 2 input neurons, 3 hidden neurons, and 4 output neurons. Furthermore, we propose the multi-layer perceptron (MLP) by utilizing analog perceptron where the structure and neurons as well as weights can be flexibly configured. The results from the measurement convinces us that our implementation attains the correct function and good performance. This paper presents the measured silicon results for the analog perceptron circuits fabricated in a 0.6 μm/☒.5 V complementary metal oxide semiconductor (CMOS) process, which are comprised of digital-to-analog converter (DAC)-based multipliers and phase shifters.
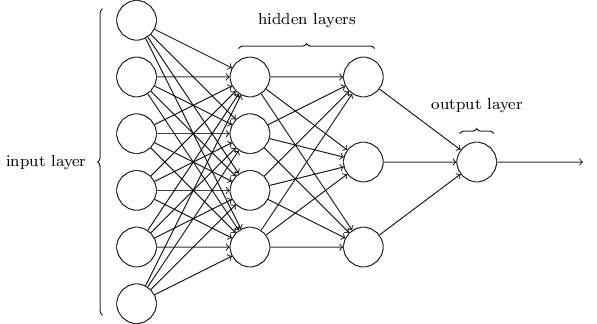
Perceptron is an essential element in neural network (NN)-based machine learning, however, the effectiveness of various implementations by circuits is rarely demonstrated from chip testing.
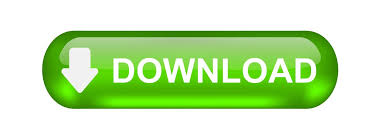